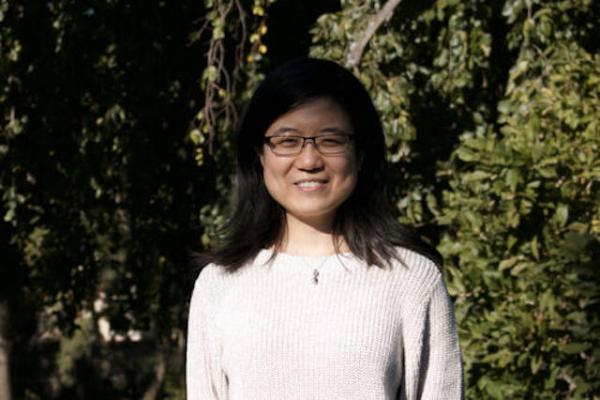
Speaker: Xinyi Chen (Yale)
Probing primordial non-Gaussianity by reconstructing the initial conditions with machine learning
Inflation remains one of the enigmas in fundamental physics. While it is difficult to distinguish different inflation models, information contained in primordial non-Gaussianity (PNG) offers a route to break the degeneracy. In galaxy surveys, the local type PNG is usually probed by measuring the scale-dependent bias in the power spectrum on large scales, where cosmic variance is also large. I will introduce a new approach to measure the local type PNG (and also other types) by using the reconstructed density field, a density field reversed to the initial conditions from late time. With the reconstructed density field, we can fit a new template at the field level, or compute a near optimal bispectrum estimator, to constrain PNG. This new approach offers an alternative way to the existing method with different systematics and also follows organically the procedure of baryon acoustic oscillation (BAO) analysis in large galaxy surveys. By reconstructing the initial conditions, we remove the nonlinearity induced by gravity, which is a source of confusion when measuring PNG. I will present a reconstruction method using convolutional neural networks that significantly improves the performance of traditional reconstruction algorithms in the matter density field, which is crucial for more effectively probing PNG. This pipeline can enable new observational constraints on PNG from the ongoing Dark Energy Spectroscopic Instrument (DESI) and Euclid surveys, as well as from upcoming surveys, such as that of the Nancy Grace Roman Space Telescope.