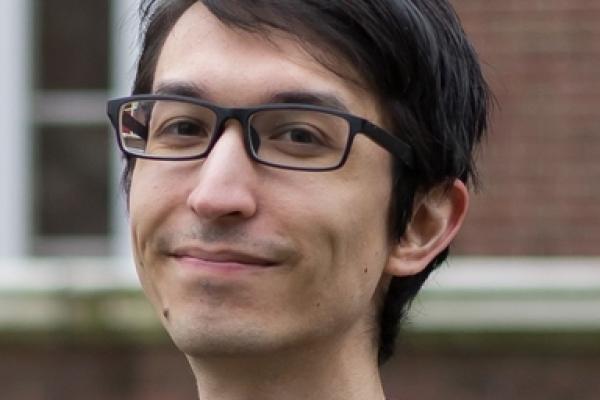
Speaker: Joshua Speagle (UToronto)
I spy with my (little?) AI: Applications of Statistical Learning in Modern Astrophysics
As datasets continue to grow, machine learning/artificial intelligence (ML/AI) has taken on an increasingly large role in scientific analyses as both a practical necessity (to handle the data volumes) but also as a way to "bypass" theoretical models by learning directly from the data. However, this speed, complexity, and flexibility also have proved to be one of the main challenges involved in actually getting scientists to "trust" the results from these ML/AI algorithms and commonly serves as a roadblock for incorporating them into a broader statistical analysis framework. In this talk, I will present a "measured" (pun intended) approach to these problems by focusing on how we can best adapt some of these approaches into a rigorous astrostatistics framework. As time permits, this will hopefully include an introduction to some of the core concepts behind the "unreasonable effectiveness" of deep learning, highlighting new results related to the scalability of these models within astrophysics, and discussing what exactly "errors" mean from these models (and how to properly incorporate/calibrate them). These will all be motivated using specific astrophysical applications, including galaxy morphology classification from broadband images, stellar parameter recovery from Gaia XP spectra, searching for extra-tidal stars in clusters, and gyrochronology of low-mass stars.